Synthetic Symbols
Generative Desires, Spare's Sigilisation Techniques and Imprints onto the Latent Space
Friends,
we’re so back. This article is one of those totally unplanned pieces written in a single take right after waking up. I’ve been thinking about building Synthetic Symbols within the Diffusion models and their resonances with Austin Osman Spare’s sigil method for quite a while, so maybe the concoction finally brewed in my deep sleep last night. I won’t be bothered with much editing, as I have a busy week, so please bear with me. Just think of this as a musing over a cup of coffee during a smoke break or sth’
Sigils and Symbols
I’ve just recently received a beautiful, and utterly bleak tarot deck designed by Austin Osman Spare from the Strange Attractor’s Kickstarter campaign. He designed the deck as tool for giving readings to his working-class clients, in the harsh conditions of early 20th-century war-torn London. Every card has inscribed little practical notes for referencing other cards in the deck, retreat with ♣3 means quarelling, with ♠7 means gossip and with 3 knights means lawsuit. Yippee-fucking-yay. The readings delivered in a mammoth spread of over two dozen cards laid out on a table, were all well… slightly sinister, to put it nicely. (No seriously, I love the deck)
And since I have this deck sitting on my table, my mind kept circling around Austin Osman Spare. A fascinating character, he has been on my radar ever since I downed his amazing and extremely readable biography by Phil Baker (with a foreword by Alan Moore). I really don’t think that his sigilisation technique, used and abused all over the contemporary magickal world needs much explanation to my dear readers, but just for the records, let’s run through the basic principles, so we can seamlessly shift into the weirder technology areas.
AOS developed a method to encode desires into symbols, supposedly a form suitable for the unconscious mind to interpret. He suggested rearranging and distilling elements of intention - spelt out as words, letters, even abstract forms—into a magickal glyph, stripped of its rational narrative context. Each fragment of the original intention gets embedded within the sigil as a carrier of the desire, consequently impressed upon one's unconscious through the process of charging/ casting. These sigils engage the deeper, instinctual layers of the psyche, translating a rationally formulated desire into a sort-of magickally charged program, executing itself within the unconscious (and/or the external world - that depends on your occult metaphysics, but that’s for another time).
Encoders and Decoders
Now, as per tradition, let’s keep these magickal terms in mind and enter into the strange world of AI. When Language Models models create semantic representations from their training data, they spatially re-construct language into a multi-dimensional space, where the word’s position encodes its meaning. I’ve spoke of these spaces called word embeddings in the previous article in quite a detail (please give it a read if you’re interested in a more in-depth intuitive explanation). This is still honestly so mindblowing to me, that I have to emphasize it again: we now have a method to work with the meaning and nuance mathematically. But keeping the exciting implications aside, let’s focus on one statement: in large language models, word embeddings are a method of translating letter-based words into a machine-understandable form, the mythical Latent Space that preserves certain semantic relations.
Diffusive AI models like Stable Diffusion or DALL-E , which transform and regenerate visual information, operate similarly. The whole process is a bit more complicated and requires a few more steps to consider, but let’s focus on the parts that are fully conceptually aligned. The basic architecture of a diffuser consists of two main components: encoder and decoder. Encoder is a part of the diffuser model, that similarly1 to word embeddings, translates the pixel space of an image into a multidimensional vector space that compresses, preserves and extracts its semantic components.
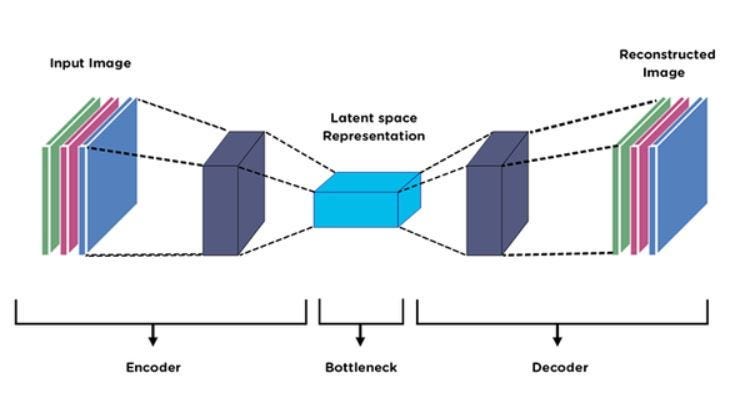
So when you input an image into an encoder, it compresses it into the latent space vector representing its key features such as texture, colour, shape and spatial configuration. Between the input image and this representation is a difference in kind, the image is translated into a mathematical construct - it doesn’t have a visual form anymore. It’s been turned into highly compressed information and we will need a decoder to translate this vector back into pixels. Think of dealing with a ‘blueprint’ of an image - in this high-dimensional space, even slight variations in the encoding can generate significant differences in the visual output. Think how even minimal defective replication of a DNA pattern might result in significant birth defects or death.
Image Injections
Training these encoder-decoder pairs, and thus obtaining the so-called foundation models is quite an undertaking. These are mostly released by major players in the field, multi-million corporations (about to destroy the planet? ehm ehm), because the training is extremely resource intensive and requires huge datasets. This is because the models need to learn the very basics of spatial perception, building from the bottom - the deepest layers of the model will encode basic geometrical representations and perspective, then they learn to distinguish colours and textures, recognise objects and progress all the way to the top layers encoding the final aesthetic touches such as photographic lenses, lighting subtleties or colourisation.
While re-training the whole model from scratch is not really feasible for an individual, there are many methods to cheaply tweak the outputs of these pre-trained models. Many techniques can be used to change or enrich the latent space, varying in their ‘invasiveness’ into the model.
Do you remember all those apps that create your tacky AI avatar after uploading half a dozen pictures of your face? That’s utilising one of the methods called Dreambooth. It is essentially a way how to (shallowly) insert a new object into the latent space so that the model can easily reference it in the future (learning who person-Karin-Valis is when prompted). This ensures that even though the object was not part of the original training data, it can be interpreted and manipulated by the model similarly to other known objects. All yours in a few minutes on a solid industrial-grade GPU.
I can’t say that the possibility of generating my own fake holiday pictures excites me much, but as always, things get interesting once you try to break them. How about, instead of using half a dozen selfies, I used seemingly random pictures encoding my desire? What will the model, attempting to extract the common representation from the input do? I feed it splinters, found and recorded, and train a deeply encoded representation of my sigil that can be referenced as an object. The model now understands this meta-structure and can generate infinite visual representations of this encoded sigil.
Look at the image above, a silly simple experiment of how this might look. I used a slightly different technique here (Textual Inversion), but the principle is similar - the input representation of whatever aspects of the ‘spell’ I’m working on (this is just a randomly assembled example for demonstration, not my real magickal work). The trained reference can be then queried through the model, and an (almost) infinite amount of visual assets can be produced. The images, generated on the right, all somehow carry the essence of the inputs, but in an abstract form, through feeling and vibe rather than strictly rational representation.
Do you feel the deep resonance with the sigil and cut-up techniques? We take the building blocks of our intent, and through the process of encoding - cutting up/ re-assembling/ re-arranging - we strip the images of their original form, yielding a new representation of our desire. This generative form is then embedded in the mind, with the ability to produce images (thoughts? visions? dreams?) understandable to the unconscious, steering us towards our desire. This runs in a deep parallel to the Synthetic Symbols that can be imprinted into the Latent Space - an encoded image, trained into the diffusion model has the ability to produce an infinite number of representations relating to the original desire. The newly formed images are inherently magickal in that they operate beyond the rational mind’s direct comprehension. They are tuned to the subtler spheres of the psyche, engaging with our unconscious mind.
Once again, it seems that the machine learning algorithms strangely resonate with well-established magickal patterns and procedures. A machinic alphabet of desire might be all vectors, but the intent remains.
Friends, thank you for staying with me.
Hope you enjoyed this essay, please share your thoughts with me. ❤️
And stay kind,
k
Further reading On Word Embeddings and the Semantic Spaces:
yes BUT ofc
Great read! btw, you might enjoy Michael Levin's recent paper - "Self-Improvising Memory: A Perspective on Memories as Agential, Dynamically Reinterpreting Cognitive Glue." He proposes a theory of organism memory based on the encoder-decoder architecture (ie, "Bow-tie").
https://www.mdpi.com/1099-4300/26/6/481